优化 (scipy.optimize
)#
scipy.optimize
包提供了几种常用的优化算法。详细列表请见: scipy.optimize
(也可以通过 help(scipy.optimize)
找到)。
多元标量函数的局部最小化 (minimize
)#
minimize
函数为 scipy.optimize
中多元标量函数的无约束和约束最小化算法提供了通用接口。为了演示最小化函数,考虑最小化 \(N\) 个变量的 Rosenbrock 函数的问题
此函数的最小值是 0,当 \(x_{i}=1.\) 时达到。
请注意,Rosenbrock 函数及其导数包含在 scipy.optimize
中。以下各节中所示的实现提供了如何定义目标函数及其雅可比矩阵和黑塞矩阵函数的示例。scipy.optimize
中的目标函数期望一个 numpy 数组作为其要优化的第一个参数,并且必须返回一个浮点值。确切的调用签名必须是 f(x, *args)
,其中 x
表示一个 numpy 数组,而 args
是传递给目标函数的附加参数的元组。
无约束最小化#
Nelder-Mead 单纯形算法 (method='Nelder-Mead'
)#
在下面的示例中,minimize
例程与 Nelder-Mead 单纯形算法一起使用 (通过 method
参数选择)
>>> import numpy as np
>>> from scipy.optimize import minimize
>>> def rosen(x):
... """The Rosenbrock function"""
... return sum(100.0*(x[1:]-x[:-1]**2.0)**2.0 + (1-x[:-1])**2.0)
>>> x0 = np.array([1.3, 0.7, 0.8, 1.9, 1.2])
>>> res = minimize(rosen, x0, method='nelder-mead',
... options={'xatol': 1e-8, 'disp': True})
Optimization terminated successfully.
Current function value: 0.000000
Iterations: 339
Function evaluations: 571
>>> print(res.x)
[1. 1. 1. 1. 1.]
单纯形算法可能是最小化行为良好的函数的最简单方法。它只需要函数求值,是简单最小化问题的不错选择。但是,由于它不使用任何梯度求值,因此可能需要更长的时间才能找到最小值。
另一个只需要函数调用即可找到最小值的优化算法是 Powell 方法,可通过在 minimize
中设置 method='powell'
来获得。
为了演示如何向目标函数提供附加参数,让我们使用附加的缩放因子 a 和偏移量 b 来最小化 Rosenbrock 函数
再次使用 minimize
例程,可以使用以下代码块针对示例参数 a=0.5
和 b=1
求解。
>>> def rosen_with_args(x, a, b):
... """The Rosenbrock function with additional arguments"""
... return sum(a*(x[1:]-x[:-1]**2.0)**2.0 + (1-x[:-1])**2.0) + b
>>> x0 = np.array([1.3, 0.7, 0.8, 1.9, 1.2])
>>> res = minimize(rosen_with_args, x0, method='nelder-mead',
... args=(0.5, 1.), options={'xatol': 1e-8, 'disp': True})
Optimization terminated successfully.
Current function value: 1.000000
Iterations: 319 # may vary
Function evaluations: 525 # may vary
>>> print(res.x)
[1. 1. 1. 1. 0.99999999]
作为使用 minimize
的 args
参数的替代方法,只需将目标函数包装在一个仅接受 x
的新函数中即可。当需要将其他参数作为关键字参数传递给目标函数时,此方法也很有用。
>>> def rosen_with_args(x, a, *, b): # b is a keyword-only argument
... return sum(a*(x[1:]-x[:-1]**2.0)**2.0 + (1-x[:-1])**2.0) + b
>>> def wrapped_rosen_without_args(x):
... return rosen_with_args(x, 0.5, b=1.) # pass in `a` and `b`
>>> x0 = np.array([1.3, 0.7, 0.8, 1.9, 1.2])
>>> res = minimize(wrapped_rosen_without_args, x0, method='nelder-mead',
... options={'xatol': 1e-8,})
>>> print(res.x)
[1. 1. 1. 1. 0.99999999]
另一种方法是使用 functools.partial
。
>>> from functools import partial
>>> partial_rosen = partial(rosen_with_args, a=0.5, b=1.)
>>> res = minimize(partial_rosen, x0, method='nelder-mead',
... options={'xatol': 1e-8,})
>>> print(res.x)
[1. 1. 1. 1. 0.99999999]
Broyden-Fletcher-Goldfarb-Shanno 算法 (method='BFGS'
)#
为了更快地收敛到解,此例程使用目标函数的梯度。如果用户没有给出梯度,则使用一阶差分进行估计。即使必须估计梯度,Broyden-Fletcher-Goldfarb-Shanno (BFGS) 方法通常也比单纯形算法需要更少的函数调用。
为了演示此算法,再次使用 Rosenbrock 函数。Rosenbrock 函数的梯度是向量
此表达式对内部导数有效。特殊情况是
使用以下代码片段构造一个计算此梯度的 Python 函数
>>> def rosen_der(x):
... xm = x[1:-1]
... xm_m1 = x[:-2]
... xm_p1 = x[2:]
... der = np.zeros_like(x)
... der[1:-1] = 200*(xm-xm_m1**2) - 400*(xm_p1 - xm**2)*xm - 2*(1-xm)
... der[0] = -400*x[0]*(x[1]-x[0]**2) - 2*(1-x[0])
... der[-1] = 200*(x[-1]-x[-2]**2)
... return der
此梯度信息通过 jac
参数在 minimize
函数中指定,如下所示。
>>> res = minimize(rosen, x0, method='BFGS', jac=rosen_der,
... options={'disp': True})
Optimization terminated successfully.
Current function value: 0.000000
Iterations: 25 # may vary
Function evaluations: 30
Gradient evaluations: 30
>>> res.x
array([1., 1., 1., 1., 1.])
避免冗余计算
目标函数及其梯度通常会共享部分计算。例如,考虑以下问题。
>>> def f(x):
... return -expensive(x[0])**2
>>>
>>> def df(x):
... return -2 * expensive(x[0]) * dexpensive(x[0])
>>>
>>> def expensive(x):
... # this function is computationally expensive!
... expensive.count += 1 # let's keep track of how many times it runs
... return np.sin(x)
>>> expensive.count = 0
>>>
>>> def dexpensive(x):
... return np.cos(x)
>>>
>>> res = minimize(f, 0.5, jac=df)
>>> res.fun
-0.9999999999999174
>>> res.nfev, res.njev
6, 6
>>> expensive.count
12
在此,expensive
被调用 12 次:在目标函数中调用 6 次,在梯度中调用 6 次。减少冗余计算的一种方法是创建一个返回目标函数和梯度的单个函数。
>>> def f_and_df(x):
... expensive_value = expensive(x[0])
... return (-expensive_value**2, # objective function
... -2*expensive_value*dexpensive(x[0])) # gradient
>>>
>>> expensive.count = 0 # reset the counter
>>> res = minimize(f_and_df, 0.5, jac=True)
>>> res.fun
-0.9999999999999174
>>> expensive.count
6
当我们调用 minimize 时,我们指定 jac==True
来表明提供的函数返回目标函数及其梯度。虽然方便,但并非所有 scipy.optimize
函数都支持此功能,而且它仅用于在函数及其梯度之间共享计算,而在某些问题中,我们希望与 Hessian(目标函数的二阶导数)和约束共享计算。一种更通用的方法是将计算的昂贵部分记忆化。在简单情况下,可以使用 functools.lru_cache
包装器来完成此操作。
>>> from functools import lru_cache
>>> expensive.count = 0 # reset the counter
>>> expensive = lru_cache(expensive)
>>> res = minimize(f, 0.5, jac=df)
>>> res.fun
-0.9999999999999174
>>> expensive.count
6
牛顿共轭梯度算法 (method='Newton-CG'
)#
牛顿共轭梯度算法是一种改进的牛顿法,它使用共轭梯度算法来(近似地)反转局部 Hessian [NW]。牛顿法基于将函数局部拟合为二次形式
其中 \(\mathbf{H}\left(\mathbf{x}_{0}\right)\) 是二阶导数矩阵(Hessian)。如果 Hessian 是正定的,则可以通过将二次形式的梯度设置为零来找到此函数的局部最小值,从而得到
Hessian 的逆是使用共轭梯度方法计算的。下面给出了使用此方法最小化 Rosenbrock 函数的示例。要充分利用 Newton-CG 方法,必须提供一个计算 Hessian 的函数。Hessian 矩阵本身不需要构造,只需要一个向量,该向量是 Hessian 与任意向量的乘积,可用于最小化例程。因此,用户可以提供一个函数来计算 Hessian 矩阵,也可以提供一个函数来计算 Hessian 与任意向量的乘积。
完整 Hessian 示例
Rosenbrock 函数的 Hessian 为
如果 \(i,j\in\left[1,N-2\right]\),其中 \(i,j\in\left[0,N-1\right]\) 定义 \(N\times N\) 矩阵。该矩阵的其他非零项是
例如,当 \(N=5\) 时,Hessian 为
以下示例显示了计算此 Hessian 的代码以及使用 Newton-CG 方法最小化函数的代码
>>> def rosen_hess(x):
... x = np.asarray(x)
... H = np.diag(-400*x[:-1],1) - np.diag(400*x[:-1],-1)
... diagonal = np.zeros_like(x)
... diagonal[0] = 1200*x[0]**2-400*x[1]+2
... diagonal[-1] = 200
... diagonal[1:-1] = 202 + 1200*x[1:-1]**2 - 400*x[2:]
... H = H + np.diag(diagonal)
... return H
>>> res = minimize(rosen, x0, method='Newton-CG',
... jac=rosen_der, hess=rosen_hess,
... options={'xtol': 1e-8, 'disp': True})
Optimization terminated successfully.
Current function value: 0.000000
Iterations: 19 # may vary
Function evaluations: 22
Gradient evaluations: 19
Hessian evaluations: 19
>>> res.x
array([1., 1., 1., 1., 1.])
Hessian 乘积示例
对于较大的最小化问题,存储整个 Hessian 矩阵会消耗大量的时间和内存。Newton-CG 算法只需要 Hessian 与任意向量的乘积。因此,用户可以通过提供一个 hess
函数来计算此乘积,而不是完整的 Hessian,该函数将最小化向量作为第一个参数,将任意向量作为第二个参数(以及传递给要最小化的函数的额外参数)。如果可能,使用带有 Hessian 乘积选项的 Newton-CG 可能是最小化函数的最快方法。
在这种情况下,Rosenbrock Hessian 与任意向量的乘积并不难计算。如果 \(\mathbf{p}\) 是任意向量,则 \(\mathbf{H}\left(\mathbf{x}\right)\mathbf{p}\) 的元素为
以下代码使用此 Hessian 乘积通过 minimize
来最小化 Rosenbrock 函数
>>> def rosen_hess_p(x, p):
... x = np.asarray(x)
... Hp = np.zeros_like(x)
... Hp[0] = (1200*x[0]**2 - 400*x[1] + 2)*p[0] - 400*x[0]*p[1]
... Hp[1:-1] = -400*x[:-2]*p[:-2]+(202+1200*x[1:-1]**2-400*x[2:])*p[1:-1] \
... -400*x[1:-1]*p[2:]
... Hp[-1] = -400*x[-2]*p[-2] + 200*p[-1]
... return Hp
>>> res = minimize(rosen, x0, method='Newton-CG',
... jac=rosen_der, hessp=rosen_hess_p,
... options={'xtol': 1e-8, 'disp': True})
Optimization terminated successfully.
Current function value: 0.000000
Iterations: 20 # may vary
Function evaluations: 23
Gradient evaluations: 20
Hessian evaluations: 44
>>> res.x
array([1., 1., 1., 1., 1.])
根据 [NW] 第 170 页,当 Hessian 条件不良时,Newton-CG
算法可能会效率低下,因为该方法在这些情况下提供的搜索方向质量较差。根据作者的说法,trust-ncg
方法可以更有效地处理这种问题,接下来将对此进行描述。
信赖域牛顿共轭梯度算法 (method='trust-ncg'
)#
Newton-CG
方法是一种线搜索方法:它找到一个搜索方向,使函数的二次近似最小化,然后使用线搜索算法找到该方向上的(几乎)最佳步长。另一种方法是,首先,固定步长限制 \(\Delta\),然后通过解决以下二次子问题来找到给定信赖半径内的最佳步长 \(\mathbf{p}\)
然后更新解 \(\mathbf{x}_{k+1} = \mathbf{x}_{k} + \mathbf{p}\),并根据二次模型与实际函数的匹配程度调整信赖半径 \(\Delta\)。这类方法称为信赖域方法。trust-ncg
算法是一种信赖域方法,它使用共轭梯度算法来解决信赖域子问题 [NW]。
完整 Hessian 示例
>>> res = minimize(rosen, x0, method='trust-ncg',
... jac=rosen_der, hess=rosen_hess,
... options={'gtol': 1e-8, 'disp': True})
Optimization terminated successfully.
Current function value: 0.000000
Iterations: 20 # may vary
Function evaluations: 21
Gradient evaluations: 20
Hessian evaluations: 19
>>> res.x
array([1., 1., 1., 1., 1.])
Hessian 乘积示例
>>> res = minimize(rosen, x0, method='trust-ncg',
... jac=rosen_der, hessp=rosen_hess_p,
... options={'gtol': 1e-8, 'disp': True})
Optimization terminated successfully.
Current function value: 0.000000
Iterations: 20 # may vary
Function evaluations: 21
Gradient evaluations: 20
Hessian evaluations: 0
>>> res.x
array([1., 1., 1., 1., 1.])
信赖域截断广义兰索斯/共轭梯度算法 (method='trust-krylov'
)#
与 trust-ncg
方法类似,trust-krylov
方法也适用于大型问题,因为它仅通过矩阵向量乘积将 Hessian 用作线性算子。它比 trust-ncg
方法更准确地解决二次子问题。
此方法包装 [TRLIB] 中 [GLTR] 方法的实现,该方法精确求解限制在截断 Krylov 子空间中的信赖域子问题。对于不定问题,通常最好使用此方法,因为它减少了非线性迭代的次数,但与 trust-ncg
方法相比,每次子问题求解的矩阵向量乘积略多。
完整 Hessian 示例
>>> res = minimize(rosen, x0, method='trust-krylov',
... jac=rosen_der, hess=rosen_hess,
... options={'gtol': 1e-8, 'disp': True})
Optimization terminated successfully.
Current function value: 0.000000
Iterations: 19 # may vary
Function evaluations: 20
Gradient evaluations: 20
Hessian evaluations: 18
>>> res.x
array([1., 1., 1., 1., 1.])
Hessian 乘积示例
>>> res = minimize(rosen, x0, method='trust-krylov',
... jac=rosen_der, hessp=rosen_hess_p,
... options={'gtol': 1e-8, 'disp': True})
Optimization terminated successfully.
Current function value: 0.000000
Iterations: 19 # may vary
Function evaluations: 20
Gradient evaluations: 20
Hessian evaluations: 0
>>> res.x
array([1., 1., 1., 1., 1.])
F. Lenders, C. Kirches, A. Potschka: “trlib: A vector-free implementation of the GLTR method for iterative solution of the trust region problem”, arXiv:1611.04718
N. Gould, S. Lucidi, M. Roma, P. Toint: “Solving the Trust-Region Subproblem using the Lanczos Method”, SIAM J. Optim., 9(2), 504–525, (1999). DOI:10.1137/S1052623497322735
信赖域近乎精确算法 (method='trust-exact'
)#
所有方法 Newton-CG
、trust-ncg
和 trust-krylov
都适用于处理大规模问题(具有数千个变量的问题)。这是因为共轭梯度算法通过迭代近似求解信赖域子问题(或反转 Hessian),而无需显式的 Hessian 分解。由于只需要 Hessian 与任意向量的乘积,因此该算法特别适合处理稀疏 Hessian,从而降低存储要求并为这些稀疏问题节省大量时间。
对于中等规模的问题,如果 Hessian 矩阵的存储和分解成本不是关键因素,可以通过几乎精确地求解信赖域子问题,在更少的迭代次数内获得解。为了实现这一点,对于每个二次子问题,会迭代求解一个特定的非线性方程 [CGT]。此解决方案通常需要对 Hessian 矩阵进行 3 或 4 次 Cholesky 分解。因此,该方法比其他已实现的信赖域方法收敛所需的迭代次数更少,并且目标函数的评估次数也更少。此算法不支持 Hessian 矩阵的乘积选项。下面是使用 Rosenbrock 函数的示例。
>>> res = minimize(rosen, x0, method='trust-exact',
... jac=rosen_der, hess=rosen_hess,
... options={'gtol': 1e-8, 'disp': True})
Optimization terminated successfully.
Current function value: 0.000000
Iterations: 13 # may vary
Function evaluations: 14
Gradient evaluations: 13
Hessian evaluations: 14
>>> res.x
array([1., 1., 1., 1., 1.])
约束最小化#
minimize
函数为约束最小化提供了几种算法,即 'trust-constr'
、'SLSQP'
、'COBYLA'
和 'COBYQA'
。它们要求使用略有不同的结构定义约束。'trust-constr'
和 'COBYQA'
方法要求将约束定义为 LinearConstraint
和 NonlinearConstraint
对象的序列。另一方面,'SLSQP'
和 'COBYLA'
方法要求将约束定义为字典序列,其中包含键 type
、fun
和 jac
。
例如,让我们考虑 Rosenbrock 函数的约束最小化
此优化问题的唯一解为 \([x_0, x_1] = [0.4149,~ 0.1701]\),其中只有第一个和第四个约束是有效的。
信赖域约束算法 (method='trust-constr'
)#
信赖域约束方法处理以下形式的约束最小化问题
当 \(c^l_j = c^u_j\) 时,该方法将第 \(j\) 个约束视为等式约束并进行相应处理。此外,可以通过将上限或下限设置为具有适当符号的 np.inf
来指定单边约束。
该实现基于用于等式约束问题的 [EQSQP] 和用于不等式约束问题的 [TRIP]。两者都是适用于大规模问题的信赖域类型算法。
定义边界约束
使用 Bounds
对象定义边界约束 \(0 \leq x_0 \leq 1\) 和 \(-0.5 \leq x_1 \leq 2.0\)。
>>> from scipy.optimize import Bounds
>>> bounds = Bounds([0, -0.5], [1.0, 2.0])
定义线性约束
约束 \(x_0 + 2 x_1 \leq 1\) 和 \(2 x_0 + x_1 = 1\) 可以用线性约束标准格式表示
并使用 LinearConstraint
对象定义。
>>> from scipy.optimize import LinearConstraint
>>> linear_constraint = LinearConstraint([[1, 2], [2, 1]], [-np.inf, 1], [1, 1])
定义非线性约束 非线性约束
其雅可比矩阵为
以及 Hessian 矩阵的线性组合为
使用 NonlinearConstraint
对象定义。
>>> def cons_f(x):
... return [x[0]**2 + x[1], x[0]**2 - x[1]]
>>> def cons_J(x):
... return [[2*x[0], 1], [2*x[0], -1]]
>>> def cons_H(x, v):
... return v[0]*np.array([[2, 0], [0, 0]]) + v[1]*np.array([[2, 0], [0, 0]])
>>> from scipy.optimize import NonlinearConstraint
>>> nonlinear_constraint = NonlinearConstraint(cons_f, -np.inf, 1, jac=cons_J, hess=cons_H)
或者,也可以将 Hessian 矩阵 \(H(x, v)\) 定义为稀疏矩阵,
>>> from scipy.sparse import csc_matrix
>>> def cons_H_sparse(x, v):
... return v[0]*csc_matrix([[2, 0], [0, 0]]) + v[1]*csc_matrix([[2, 0], [0, 0]])
>>> nonlinear_constraint = NonlinearConstraint(cons_f, -np.inf, 1,
... jac=cons_J, hess=cons_H_sparse)
或定义为 LinearOperator
对象。
>>> from scipy.sparse.linalg import LinearOperator
>>> def cons_H_linear_operator(x, v):
... def matvec(p):
... return np.array([p[0]*2*(v[0]+v[1]), 0])
... return LinearOperator((2, 2), matvec=matvec)
>>> nonlinear_constraint = NonlinearConstraint(cons_f, -np.inf, 1,
... jac=cons_J, hess=cons_H_linear_operator)
当 Hessian 矩阵 \(H(x, v)\) 的计算难以实现或在计算上不可行时,可以使用 HessianUpdateStrategy
。目前可用的策略是 BFGS
和 SR1
。
>>> from scipy.optimize import BFGS
>>> nonlinear_constraint = NonlinearConstraint(cons_f, -np.inf, 1, jac=cons_J, hess=BFGS())
或者,可以使用有限差分来近似 Hessian 矩阵。
>>> nonlinear_constraint = NonlinearConstraint(cons_f, -np.inf, 1, jac=cons_J, hess='2-point')
约束的雅可比矩阵也可以通过有限差分来近似。然而,在这种情况下,Hessian 矩阵无法通过有限差分计算,需要由用户提供或使用 HessianUpdateStrategy
定义。
>>> nonlinear_constraint = NonlinearConstraint(cons_f, -np.inf, 1, jac='2-point', hess=BFGS())
求解优化问题 优化问题使用以下方法求解
>>> x0 = np.array([0.5, 0])
>>> res = minimize(rosen, x0, method='trust-constr', jac=rosen_der, hess=rosen_hess,
... constraints=[linear_constraint, nonlinear_constraint],
... options={'verbose': 1}, bounds=bounds)
# may vary
`gtol` termination condition is satisfied.
Number of iterations: 12, function evaluations: 8, CG iterations: 7, optimality: 2.99e-09, constraint violation: 1.11e-16, execution time: 0.016 s.
>>> print(res.x)
[0.41494531 0.17010937]
如果需要,可以使用 LinearOperator
对象定义目标函数的 Hessian 矩阵,
>>> def rosen_hess_linop(x):
... def matvec(p):
... return rosen_hess_p(x, p)
... return LinearOperator((2, 2), matvec=matvec)
>>> res = minimize(rosen, x0, method='trust-constr', jac=rosen_der, hess=rosen_hess_linop,
... constraints=[linear_constraint, nonlinear_constraint],
... options={'verbose': 1}, bounds=bounds)
# may vary
`gtol` termination condition is satisfied.
Number of iterations: 12, function evaluations: 8, CG iterations: 7, optimality: 2.99e-09, constraint violation: 1.11e-16, execution time: 0.018 s.
>>> print(res.x)
[0.41494531 0.17010937]
或者通过参数 hessp
定义 Hessian 矩阵-向量积。
>>> res = minimize(rosen, x0, method='trust-constr', jac=rosen_der, hessp=rosen_hess_p,
... constraints=[linear_constraint, nonlinear_constraint],
... options={'verbose': 1}, bounds=bounds)
# may vary
`gtol` termination condition is satisfied.
Number of iterations: 12, function evaluations: 8, CG iterations: 7, optimality: 2.99e-09, constraint violation: 1.11e-16, execution time: 0.018 s.
>>> print(res.x)
[0.41494531 0.17010937]
或者,可以近似目标函数的一阶和二阶导数。例如,可以使用 SR1
拟牛顿近似来近似 Hessian 矩阵,并使用有限差分近似梯度。
>>> from scipy.optimize import SR1
>>> res = minimize(rosen, x0, method='trust-constr', jac="2-point", hess=SR1(),
... constraints=[linear_constraint, nonlinear_constraint],
... options={'verbose': 1}, bounds=bounds)
# may vary
`gtol` termination condition is satisfied.
Number of iterations: 12, function evaluations: 24, CG iterations: 7, optimality: 4.48e-09, constraint violation: 0.00e+00, execution time: 0.016 s.
>>> print(res.x)
[0.41494531 0.17010937]
Byrd, Richard H., Mary E. Hribar, and Jorge Nocedal. 1999. An interior point algorithm for large-scale nonlinear programming. SIAM Journal on Optimization 9.4: 877-900.
Lalee, Marucha, Jorge Nocedal, and Todd Plantega. 1998. On the implementation of an algorithm for large-scale equality constrained optimization. SIAM Journal on Optimization 8.3: 682-706.
序列最小二乘规划 (SLSQP) 算法 (method='SLSQP'
)#
SLSQP 方法处理以下形式的约束最小化问题
其中 \(\mathcal{E}\) 或 \(\mathcal{I}\) 是包含等式和不等式约束的索引集。
线性约束和非线性约束都定义为带有键 type
、fun
和 jac
的字典。
>>> ineq_cons = {'type': 'ineq',
... 'fun' : lambda x: np.array([1 - x[0] - 2*x[1],
... 1 - x[0]**2 - x[1],
... 1 - x[0]**2 + x[1]]),
... 'jac' : lambda x: np.array([[-1.0, -2.0],
... [-2*x[0], -1.0],
... [-2*x[0], 1.0]])}
>>> eq_cons = {'type': 'eq',
... 'fun' : lambda x: np.array([2*x[0] + x[1] - 1]),
... 'jac' : lambda x: np.array([2.0, 1.0])}
优化问题使用以下方法求解
>>> x0 = np.array([0.5, 0])
>>> res = minimize(rosen, x0, method='SLSQP', jac=rosen_der,
... constraints=[eq_cons, ineq_cons], options={'ftol': 1e-9, 'disp': True},
... bounds=bounds)
# may vary
Optimization terminated successfully. (Exit mode 0)
Current function value: 0.342717574857755
Iterations: 5
Function evaluations: 6
Gradient evaluations: 5
>>> print(res.x)
[0.41494475 0.1701105 ]
方法 'trust-constr'
的大多数可用选项不适用于 'SLSQP'
。
局部最小化求解器比较#
使用下表查找满足您要求的求解器。如果有多个候选项,请尝试几个,看看哪个最能满足您的需求(例如执行时间、目标函数值)。
求解器 |
边界约束 |
非线性约束 |
使用梯度 |
使用 Hessian 矩阵 |
利用稀疏性 |
---|---|---|---|---|---|
CG |
✓ |
||||
BFGS |
✓ |
||||
dogleg |
✓ |
✓ |
|||
trust-ncg |
✓ |
✓ |
|||
trust-krylov |
✓ |
✓ |
|||
trust-exact |
✓ |
✓ |
|||
Newton-CG |
✓ |
✓ |
✓ |
||
Nelder-Mead |
✓ |
||||
Powell |
✓ |
||||
L-BFGS-B |
✓ |
✓ |
|||
TNC |
✓ |
✓ |
|||
COBYLA |
✓ |
✓ |
|||
SLSQP |
✓ |
✓ |
✓ |
||
trust-constr |
✓ |
✓ |
✓ |
✓ |
✓ |
全局优化#
全局优化旨在寻找给定边界内函数的全局最小值,在存在潜在的许多局部最小值的情况下。通常,全局最小化器会有效地搜索参数空间,同时在后台使用局部最小化器(例如,minimize
)。SciPy 包含许多优秀的全局优化器。在这里,我们将在相同的目标函数上使用它们,即(恰如其名)eggholder
函数。
>>> def eggholder(x):
... return (-(x[1] + 47) * np.sin(np.sqrt(abs(x[0]/2 + (x[1] + 47))))
... -x[0] * np.sin(np.sqrt(abs(x[0] - (x[1] + 47)))))
>>> bounds = [(-512, 512), (-512, 512)]
这个函数看起来像一个鸡蛋盒。
>>> import matplotlib.pyplot as plt
>>> from mpl_toolkits.mplot3d import Axes3D
>>> x = np.arange(-512, 513)
>>> y = np.arange(-512, 513)
>>> xgrid, ygrid = np.meshgrid(x, y)
>>> xy = np.stack([xgrid, ygrid])
>>> fig = plt.figure()
>>> ax = fig.add_subplot(111, projection='3d')
>>> ax.view_init(45, -45)
>>> ax.plot_surface(xgrid, ygrid, eggholder(xy), cmap='terrain')
>>> ax.set_xlabel('x')
>>> ax.set_ylabel('y')
>>> ax.set_zlabel('eggholder(x, y)')
>>> plt.show()
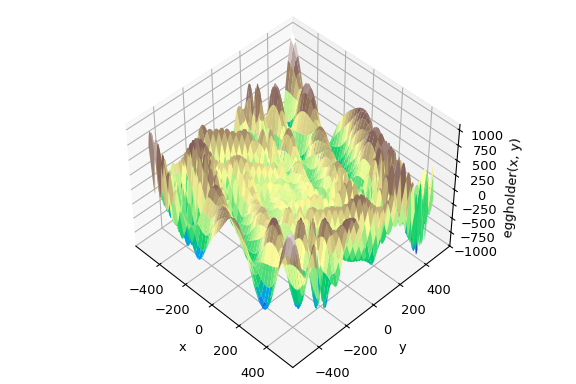
现在我们使用全局优化器来获得最小值和最小值处的函数值。我们将结果存储在一个字典中,以便稍后比较不同的优化结果。
>>> from scipy import optimize
>>> results = dict()
>>> results['shgo'] = optimize.shgo(eggholder, bounds)
>>> results['shgo']
fun: -935.3379515604197 # may vary
funl: array([-935.33795156])
message: 'Optimization terminated successfully.'
nfev: 42
nit: 2
nlfev: 37
nlhev: 0
nljev: 9
success: True
x: array([439.48096952, 453.97740589])
xl: array([[439.48096952, 453.97740589]])
>>> results['DA'] = optimize.dual_annealing(eggholder, bounds)
>>> results['DA']
fun: -956.9182316237413 # may vary
message: ['Maximum number of iteration reached']
nfev: 4091
nhev: 0
nit: 1000
njev: 0
x: array([482.35324114, 432.87892901])
所有优化器都返回一个 OptimizeResult
,除了解决方案外,还包含有关函数评估次数、优化是否成功等信息。为简洁起见,我们将不显示其他优化器的完整输出。
>>> results['DE'] = optimize.differential_evolution(eggholder, bounds)
shgo
有第二种方法,它返回所有局部最小值,而不仅仅是它认为的全局最小值。
>>> results['shgo_sobol'] = optimize.shgo(eggholder, bounds, n=200, iters=5,
... sampling_method='sobol')
现在我们将在该函数的热图上绘制所有找到的最小值。
>>> fig = plt.figure()
>>> ax = fig.add_subplot(111)
>>> im = ax.imshow(eggholder(xy), interpolation='bilinear', origin='lower',
... cmap='gray')
>>> ax.set_xlabel('x')
>>> ax.set_ylabel('y')
>>>
>>> def plot_point(res, marker='o', color=None):
... ax.plot(512+res.x[0], 512+res.x[1], marker=marker, color=color, ms=10)
>>> plot_point(results['DE'], color='c') # differential_evolution - cyan
>>> plot_point(results['DA'], color='w') # dual_annealing. - white
>>> # SHGO produces multiple minima, plot them all (with a smaller marker size)
>>> plot_point(results['shgo'], color='r', marker='+')
>>> plot_point(results['shgo_sobol'], color='r', marker='x')
>>> for i in range(results['shgo_sobol'].xl.shape[0]):
... ax.plot(512 + results['shgo_sobol'].xl[i, 0],
... 512 + results['shgo_sobol'].xl[i, 1],
... 'ro', ms=2)
>>> ax.set_xlim([-4, 514*2])
>>> ax.set_ylim([-4, 514*2])
>>> plt.show()
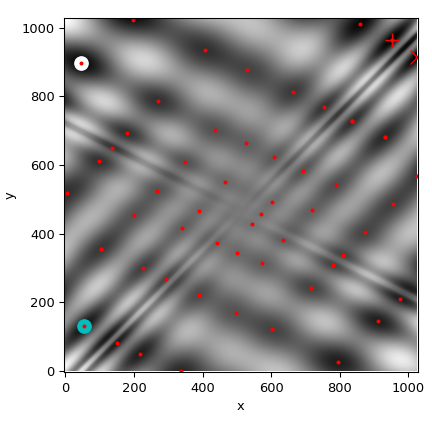
全局优化器的比较#
使用下表查找满足您要求的求解器。如果有多个候选项,请尝试几个,看看哪个最能满足您的需求(例如执行时间、目标函数值)。
求解器 |
边界约束 |
非线性约束 |
使用梯度 |
使用 Hessian 矩阵 |
---|---|---|---|---|
basinhopping |
(✓) |
(✓) |
||
direct |
✓ |
|||
dual_annealing |
✓ |
(✓) |
(✓) |
|
differential_evolution |
✓ |
✓ |
||
shgo |
✓ |
✓ |
(✓) |
(✓) |
(✓) = 取决于选择的局部最小化器
最小二乘法最小化 (least_squares
)#
SciPy 能够解决鲁棒的、带边界约束的非线性最小二乘问题。
这里 \(f_i(\mathbf{x})\) 是从 \(\mathbb{R}^n\) 到 \(\mathbb{R}\) 的平滑函数,我们称之为残差。标量值函数 \(\rho(\cdot)\) 的目的是减少离群残差的影响,并有助于解的鲁棒性,我们称之为损失函数。线性损失函数给出一个标准的最小二乘问题。此外,允许对某些 \(x_j\) 设置下限和上限形式的约束。
所有特定于最小二乘最小化的方法都使用一个称为雅可比矩阵的 \(m \times n\) 偏导数矩阵,定义为 \(J_{ij} = \partial f_i / \partial x_j\)。强烈建议以解析方式计算此矩阵并将其传递给 least_squares
,否则,它将通过有限差分来估计,这需要大量额外的时间,并且在困难的情况下可能非常不准确。
函数 least_squares
可用于将函数 \(\varphi(t; \mathbf{x})\) 拟合到经验数据 \(\{(t_i, y_i), i = 0, \ldots, m-1\}\)。为此,应该简单地预先计算残差为 \(f_i(\mathbf{x}) = w_i (\varphi(t_i; \mathbf{x}) - y_i)\),其中 \(w_i\) 是分配给每个观测值的权重。
求解拟合问题的示例#
在这里,我们考虑一个酶促反应 [1]。有 11 个残差定义为
其中 \(y_i\) 是测量值,\(u_i\) 是自变量的值。参数的未知向量是 \(\mathbf{x} = (x_0, x_1, x_2, x_3)^T\)。如前所述,建议以闭合形式计算雅可比矩阵
我们将使用 [2] 中定义的“硬”起始点。为了找到一个有物理意义的解,避免潜在的除以零并确保收敛到全局最小值,我们施加约束 \(0 \leq x_j \leq 100, j = 0, 1, 2, 3\)。
下面的代码实现了 \(\mathbf{x}\) 的最小二乘估计,最后绘制原始数据和拟合模型函数。
>>> from scipy.optimize import least_squares
>>> def model(x, u):
... return x[0] * (u ** 2 + x[1] * u) / (u ** 2 + x[2] * u + x[3])
>>> def fun(x, u, y):
... return model(x, u) - y
>>> def jac(x, u, y):
... J = np.empty((u.size, x.size))
... den = u ** 2 + x[2] * u + x[3]
... num = u ** 2 + x[1] * u
... J[:, 0] = num / den
... J[:, 1] = x[0] * u / den
... J[:, 2] = -x[0] * num * u / den ** 2
... J[:, 3] = -x[0] * num / den ** 2
... return J
>>> u = np.array([4.0, 2.0, 1.0, 5.0e-1, 2.5e-1, 1.67e-1, 1.25e-1, 1.0e-1,
... 8.33e-2, 7.14e-2, 6.25e-2])
>>> y = np.array([1.957e-1, 1.947e-1, 1.735e-1, 1.6e-1, 8.44e-2, 6.27e-2,
... 4.56e-2, 3.42e-2, 3.23e-2, 2.35e-2, 2.46e-2])
>>> x0 = np.array([2.5, 3.9, 4.15, 3.9])
>>> res = least_squares(fun, x0, jac=jac, bounds=(0, 100), args=(u, y), verbose=1)
# may vary
`ftol` termination condition is satisfied.
Function evaluations 130, initial cost 4.4383e+00, final cost 1.5375e-04, first-order optimality 4.92e-08.
>>> res.x
array([ 0.19280596, 0.19130423, 0.12306063, 0.13607247])
>>> import matplotlib.pyplot as plt
>>> u_test = np.linspace(0, 5)
>>> y_test = model(res.x, u_test)
>>> plt.plot(u, y, 'o', markersize=4, label='data')
>>> plt.plot(u_test, y_test, label='fitted model')
>>> plt.xlabel("u")
>>> plt.ylabel("y")
>>> plt.legend(loc='lower right')
>>> plt.show()
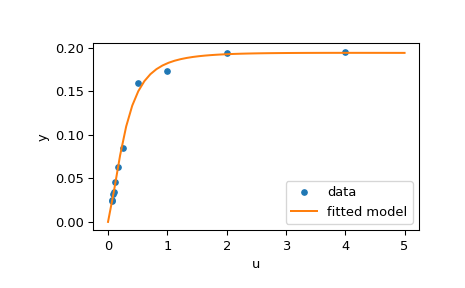
更多示例#
下面三个交互式示例更详细地说明了 least_squares
的用法。
scipy 中的大规模捆绑调整 演示了
least_squares
的大规模功能,以及如何有效地计算稀疏雅可比矩阵的有限差分近似值。scipy 中的鲁棒非线性回归 展示了如何在非线性回归中使用鲁棒损失函数来处理离群值。
在 scipy 中解决离散边界值问题 研究了如何解决大型方程组,并使用边界来实现解的期望属性。
有关实现背后数学算法的详细信息,请参阅 least_squares
的文档。
单变量函数最小化器 (minimize_scalar
)#
通常只需要单变量函数(即,将标量作为输入的函数)的最小值。在这种情况下,已经开发了其他可以更快工作的优化技术。这些可以从 minimize_scalar
函数访问,该函数提出了几种算法。
无约束最小化 (method='brent'
)#
实际上,有两种方法可以用来最小化单变量函数:brent
和 golden
,但 golden
仅用于学术目的,应很少使用。这些可以通过 minimize_scalar
中的 method 参数分别选择。 brent
方法使用 Brent 算法来定位最小值。理想情况下,应给出包含所需最小值的括号(bracket
参数)。括号是三元组 \(\left( a, b, c \right)\),使得 \(f \left( a \right) > f \left( b \right) < f \left( c \right)\) 并且 \(a < b < c\)。如果未给出此项,则可以选择两个起始点,并将使用简单的行进算法从这些点找到一个括号。如果未提供这两个起始点,则将使用 0 和 1(这可能不是您函数的正确选择,并且可能导致返回意外的最小值)。
这是一个示例
>>> from scipy.optimize import minimize_scalar
>>> f = lambda x: (x - 2) * (x + 1)**2
>>> res = minimize_scalar(f, method='brent')
>>> print(res.x)
1.0
有界最小化 (method='bounded'
)#
通常,在进行最小化之前,可以对解空间施加约束。 minimize_scalar
中的bounded 方法就是一个受约束的最小化过程的示例,它为标量函数提供了基本的区间约束。区间约束允许最小化仅在两个固定的端点之间进行,这两个端点使用强制性的bounds 参数指定。
例如,要找到 \(J_{1}\left( x \right)\) 在 \(x=5\) 附近的最小值,可以使用区间 \(\left[ 4, 7 \right]\) 作为约束调用 minimize_scalar
。结果为 \(x_{\textrm{min}}=5.3314\)
>>> from scipy.special import j1
>>> res = minimize_scalar(j1, bounds=(4, 7), method='bounded')
>>> res.x
5.33144184241
自定义最小化器#
有时,使用自定义方法作为(多元或单变量)最小化器可能很有用,例如,当使用 minimize
的某些库包装器时(例如,basinhopping
)。
我们可以通过传递一个可调用对象(函数或实现 __call__ 方法的对象)作为method 参数来实现这一点,而不是传递方法名称。
让我们考虑一个(无可否认相当虚拟的)需求,即使用一个简单的自定义多元最小化方法,该方法将仅在每个维度中独立地以固定的步长搜索邻域。
>>> from scipy.optimize import OptimizeResult
>>> def custmin(fun, x0, args=(), maxfev=None, stepsize=0.1,
... maxiter=100, callback=None, **options):
... bestx = x0
... besty = fun(x0)
... funcalls = 1
... niter = 0
... improved = True
... stop = False
...
... while improved and not stop and niter < maxiter:
... improved = False
... niter += 1
... for dim in range(np.size(x0)):
... for s in [bestx[dim] - stepsize, bestx[dim] + stepsize]:
... testx = np.copy(bestx)
... testx[dim] = s
... testy = fun(testx, *args)
... funcalls += 1
... if testy < besty:
... besty = testy
... bestx = testx
... improved = True
... if callback is not None:
... callback(bestx)
... if maxfev is not None and funcalls >= maxfev:
... stop = True
... break
...
... return OptimizeResult(fun=besty, x=bestx, nit=niter,
... nfev=funcalls, success=(niter > 1))
>>> x0 = [1.35, 0.9, 0.8, 1.1, 1.2]
>>> res = minimize(rosen, x0, method=custmin, options=dict(stepsize=0.05))
>>> res.x
array([1., 1., 1., 1., 1.])
这在单变量优化的情况下也同样适用。
>>> def custmin(fun, bracket, args=(), maxfev=None, stepsize=0.1,
... maxiter=100, callback=None, **options):
... bestx = (bracket[1] + bracket[0]) / 2.0
... besty = fun(bestx)
... funcalls = 1
... niter = 0
... improved = True
... stop = False
...
... while improved and not stop and niter < maxiter:
... improved = False
... niter += 1
... for testx in [bestx - stepsize, bestx + stepsize]:
... testy = fun(testx, *args)
... funcalls += 1
... if testy < besty:
... besty = testy
... bestx = testx
... improved = True
... if callback is not None:
... callback(bestx)
... if maxfev is not None and funcalls >= maxfev:
... stop = True
... break
...
... return OptimizeResult(fun=besty, x=bestx, nit=niter,
... nfev=funcalls, success=(niter > 1))
>>> def f(x):
... return (x - 2)**2 * (x + 2)**2
>>> res = minimize_scalar(f, bracket=(-3.5, 0), method=custmin,
... options=dict(stepsize = 0.05))
>>> res.x
-2.0
求根#
标量函数#
如果有一个单变量方程,则可以尝试多种不同的求根算法。这些算法中的大多数都需要一个区间端点,其中预计会有一个根(因为该函数会改变符号)。通常,brentq
是最佳选择,但其他方法在某些情况下或出于学术目的可能有用。当没有括号可用,但有一个或多个导数可用时,则可以使用 newton
(或 halley
,secant
)。如果该函数定义在复平面的子集上,并且无法使用括号方法,则尤其如此。
不动点求解#
与求函数的零点密切相关的一个问题是求函数不动点的问题。函数的不动点是函数求值返回该点的点: \(g\left(x\right)=x.\) 显然,\(g\) 的不动点是 \(f\left(x\right)=g\left(x\right)-x.\) 的根。等效地,\(f\) 的根是 \(g\left(x\right)=f\left(x\right)+x.\) 的不动点。例程 fixed_point
提供了一种简单的迭代方法,使用 Aitken 序列加速来估计给定起点的 \(g\) 的不动点。
方程组#
可以使用 root
函数找到一组非线性方程的根。有几种方法可用,其中包括 hybr
(默认方法)和 lm
,它们分别使用 Powell 的混合方法和 MINPACK 中的 Levenberg-Marquardt 方法。
以下示例考虑了单变量超越方程
其根可以如下找到
>>> import numpy as np
>>> from scipy.optimize import root
>>> def func(x):
... return x + 2 * np.cos(x)
>>> sol = root(func, 0.3)
>>> sol.x
array([-1.02986653])
>>> sol.fun
array([ -6.66133815e-16])
现在考虑一组非线性方程
我们定义目标函数,以便它也返回雅可比矩阵,并通过将 jac
参数设置为 True
来指示这一点。此外,这里还使用了 Levenberg-Marquardt 求解器。
>>> def func2(x):
... f = [x[0] * np.cos(x[1]) - 4,
... x[1]*x[0] - x[1] - 5]
... df = np.array([[np.cos(x[1]), -x[0] * np.sin(x[1])],
... [x[1], x[0] - 1]])
... return f, df
>>> sol = root(func2, [1, 1], jac=True, method='lm')
>>> sol.x
array([ 6.50409711, 0.90841421])
大型问题的求根#
root
中的方法 hybr
和 lm
无法处理非常多的变量 (N),因为它们需要在每个牛顿步上计算并反转密集的 N x N 雅可比矩阵。当 N 增长时,这变得相当低效。
例如,考虑以下问题:我们需要在正方形 \([0,1]\times[0,1]\) 上求解以下积分微分方程
边界条件是上边缘上的 \(P(x,1) = 1\) ,而正方形边界上的其他位置为 \(P=0\) 。可以通过用网格上的值 \(P_{n,m}\approx{}P(n h, m h)\) 来逼近连续函数 P 来完成此操作,其中网格间距 h 很小。然后可以近似导数和积分;例如 \(\partial_x^2 P(x,y)\approx{}(P(x+h,y) - 2 P(x,y) + P(x-h,y))/h^2\)。然后,该问题等效于找到某个函数 residual(P)
的根,其中 P
是长度为 \(N_x N_y\) 的向量。
现在,由于 \(N_x N_y\) 可能很大, root
中的方法 hybr
或 lm
将需要很长时间才能解决此问题。但是,可以使用大型求解器之一找到解决方案,例如 krylov
, broyden2
或 anderson
。这些使用所谓的不精确牛顿方法,该方法不是精确计算雅可比矩阵,而是为其形成近似值。
我们现在可以按如下方式解决我们遇到的问题
import numpy as np
from scipy.optimize import root
from numpy import cosh, zeros_like, mgrid, zeros
# parameters
nx, ny = 75, 75
hx, hy = 1./(nx-1), 1./(ny-1)
P_left, P_right = 0, 0
P_top, P_bottom = 1, 0
def residual(P):
d2x = zeros_like(P)
d2y = zeros_like(P)
d2x[1:-1] = (P[2:] - 2*P[1:-1] + P[:-2]) / hx/hx
d2x[0] = (P[1] - 2*P[0] + P_left)/hx/hx
d2x[-1] = (P_right - 2*P[-1] + P[-2])/hx/hx
d2y[:,1:-1] = (P[:,2:] - 2*P[:,1:-1] + P[:,:-2])/hy/hy
d2y[:,0] = (P[:,1] - 2*P[:,0] + P_bottom)/hy/hy
d2y[:,-1] = (P_top - 2*P[:,-1] + P[:,-2])/hy/hy
return d2x + d2y + 5*cosh(P).mean()**2
# solve
guess = zeros((nx, ny), float)
sol = root(residual, guess, method='krylov', options={'disp': True})
#sol = root(residual, guess, method='broyden2', options={'disp': True, 'max_rank': 50})
#sol = root(residual, guess, method='anderson', options={'disp': True, 'M': 10})
print('Residual: %g' % abs(residual(sol.x)).max())
# visualize
import matplotlib.pyplot as plt
x, y = mgrid[0:1:(nx*1j), 0:1:(ny*1j)]
plt.pcolormesh(x, y, sol.x, shading='gouraud')
plt.colorbar()
plt.show()
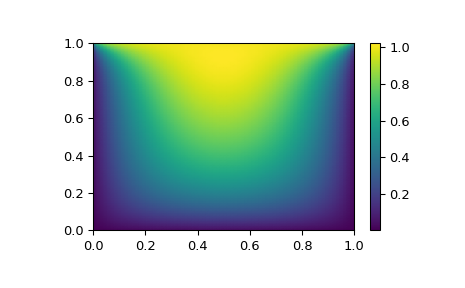
仍然太慢?预处理。#
当寻找函数 \(f_i({\bf x}) = 0\) 的零点时,i = 1, 2, …, N, krylov
求解器将大部分时间用于反转雅可比矩阵,
如果您有逆矩阵的近似值 \(M\approx{}J^{-1}\),则可以使用它来预处理线性反演问题。想法是,与其求解 \(J{\bf s}={\bf y}\),不如求解 \(MJ{\bf s}=M{\bf y}\):由于矩阵 \(MJ\) 比 \(J\) “更接近”单位矩阵,因此该方程应该更容易让 Krylov 方法处理。
矩阵 M 可以作为选项 options['jac_options']['inner_M']
传递给使用方法 krylov
的 root
。它可以是(稀疏)矩阵或 scipy.sparse.linalg.LinearOperator
实例。
对于上一节中的问题,我们注意到要解决的函数由两部分组成:第一部分是拉普拉斯算子 \([\partial_x^2 + \partial_y^2] P\)的应用,第二部分是积分。我们实际上可以轻松地计算对应于拉普拉斯算子部分的雅可比矩阵:我们知道在 1-D 中
因此,整个 2-D 运算符由下式表示
与积分对应的雅可比矩阵 \(J_2\) 更难计算,并且由于它的所有条目都是非零的,因此很难反转。另一方面,\(J_1\) 是一个相对简单的矩阵,可以使用 scipy.sparse.linalg.splu
反转(或可以使用 scipy.sparse.linalg.spilu
来逼近反转)。因此,我们乐于选择 \(M\approx{}J_1^{-1}\) 并期望结果最好。
在下面的示例中,我们使用预处理器 \(M=J_1^{-1}\)。
from scipy.optimize import root
from scipy.sparse import dia_array, kron
from scipy.sparse.linalg import spilu, LinearOperator
from numpy import cosh, zeros_like, mgrid, zeros, eye
# parameters
nx, ny = 75, 75
hx, hy = 1./(nx-1), 1./(ny-1)
P_left, P_right = 0, 0
P_top, P_bottom = 1, 0
def get_preconditioner():
"""Compute the preconditioner M"""
diags_x = zeros((3, nx))
diags_x[0,:] = 1/hx/hx
diags_x[1,:] = -2/hx/hx
diags_x[2,:] = 1/hx/hx
Lx = dia_array((diags_x, [-1,0,1]), shape=(nx, nx))
diags_y = zeros((3, ny))
diags_y[0,:] = 1/hy/hy
diags_y[1,:] = -2/hy/hy
diags_y[2,:] = 1/hy/hy
Ly = dia_array((diags_y, [-1,0,1]), shape=(ny, ny))
J1 = kron(Lx, eye(ny)) + kron(eye(nx), Ly)
# Now we have the matrix `J_1`. We need to find its inverse `M` --
# however, since an approximate inverse is enough, we can use
# the *incomplete LU* decomposition
J1_ilu = spilu(J1)
# This returns an object with a method .solve() that evaluates
# the corresponding matrix-vector product. We need to wrap it into
# a LinearOperator before it can be passed to the Krylov methods:
M = LinearOperator(shape=(nx*ny, nx*ny), matvec=J1_ilu.solve)
return M
def solve(preconditioning=True):
"""Compute the solution"""
count = [0]
def residual(P):
count[0] += 1
d2x = zeros_like(P)
d2y = zeros_like(P)
d2x[1:-1] = (P[2:] - 2*P[1:-1] + P[:-2])/hx/hx
d2x[0] = (P[1] - 2*P[0] + P_left)/hx/hx
d2x[-1] = (P_right - 2*P[-1] + P[-2])/hx/hx
d2y[:,1:-1] = (P[:,2:] - 2*P[:,1:-1] + P[:,:-2])/hy/hy
d2y[:,0] = (P[:,1] - 2*P[:,0] + P_bottom)/hy/hy
d2y[:,-1] = (P_top - 2*P[:,-1] + P[:,-2])/hy/hy
return d2x + d2y + 5*cosh(P).mean()**2
# preconditioner
if preconditioning:
M = get_preconditioner()
else:
M = None
# solve
guess = zeros((nx, ny), float)
sol = root(residual, guess, method='krylov',
options={'disp': True,
'jac_options': {'inner_M': M}})
print('Residual', abs(residual(sol.x)).max())
print('Evaluations', count[0])
return sol.x
def main():
sol = solve(preconditioning=True)
# visualize
import matplotlib.pyplot as plt
x, y = mgrid[0:1:(nx*1j), 0:1:(ny*1j)]
plt.clf()
plt.pcolor(x, y, sol)
plt.clim(0, 1)
plt.colorbar()
plt.show()
if __name__ == "__main__":
main()
执行结果,首先不进行预处理
0: |F(x)| = 803.614; step 1; tol 0.000257947
1: |F(x)| = 345.912; step 1; tol 0.166755
2: |F(x)| = 139.159; step 1; tol 0.145657
3: |F(x)| = 27.3682; step 1; tol 0.0348109
4: |F(x)| = 1.03303; step 1; tol 0.00128227
5: |F(x)| = 0.0406634; step 1; tol 0.00139451
6: |F(x)| = 0.00344341; step 1; tol 0.00645373
7: |F(x)| = 0.000153671; step 1; tol 0.00179246
8: |F(x)| = 6.7424e-06; step 1; tol 0.00173256
Residual 3.57078908664e-07
Evaluations 317
然后再进行预处理
0: |F(x)| = 136.993; step 1; tol 7.49599e-06
1: |F(x)| = 4.80983; step 1; tol 0.00110945
2: |F(x)| = 0.195942; step 1; tol 0.00149362
3: |F(x)| = 0.000563597; step 1; tol 7.44604e-06
4: |F(x)| = 1.00698e-09; step 1; tol 2.87308e-12
Residual 9.29603061195e-11
Evaluations 77
使用预处理器将 residual
函数的求值次数减少了 4 倍。对于计算成本很高的残差问题,良好的预处理至关重要——它甚至可以决定该问题在实践中是否可解。
预处理是一门艺术、科学和产业。在这里,我们很幸运地做出了一个简单但效果不错的选择,但是这个主题比这里显示的要深得多。
线性规划 (linprog
)#
函数 linprog
可以最小化受线性等式和不等式约束的线性目标函数。这类问题众所周知是线性规划。线性规划解决以下形式的问题
其中 \(x\) 是决策变量的向量; \(c\)、\(b_{ub}\)、\(b_{eq}\)、\(l\) 和 \(u\) 是向量; \(A_{ub}\) 和 \(A_{eq}\) 是矩阵。
在本教程中,我们将尝试使用 linprog
解决一个典型的线性规划问题。
线性规划示例#
考虑以下简单的线性规划问题
我们需要进行一些数学运算,将目标问题转换为 linprog
可以接受的形式。
首先,让我们考虑目标函数。 我们想要最大化目标函数,但是 linprog
只能接受最小化问题。 这可以通过将最大化 \(29x_1 + 45x_2\) 转换为最小化 \(-29x_1 -45x_2\) 来轻松解决。此外,目标函数中没有显示 \(x_3, x_4\)。这意味着与 \(x_3, x_4\) 相对应的权重为零。因此,目标函数可以转换为
如果我们定义决策变量的向量 \(x = [x_1, x_2, x_3, x_4]^T\),则此问题中 linprog
的目标权重向量 \(c\) 应为
接下来,让我们考虑两个不等式约束。第一个是“小于”不等式,因此它已经是 linprog
接受的形式。第二个是“大于”不等式,因此我们需要将两边都乘以 \(-1\) 以将其转换为“小于”不等式。显式显示零系数,我们有
这些方程可以转换为矩阵形式
其中
接下来,让我们考虑两个等式约束。显式显示零权重,这些是
这些方程可以转换为矩阵形式
其中
最后,让我们考虑对各个决策变量的单独不等式约束,这些约束被称为“盒约束”或“简单边界”。这些约束可以使用 linprog
的 bounds 参数应用。如 linprog
文档中所述,bounds 的默认值为 (0, None)
,这意味着每个决策变量的下限为 0,每个决策变量的上限为无穷大:所有决策变量都是非负的。我们的边界不同,因此我们需要将每个决策变量的下限和上限指定为元组,并将这些元组分组到一个列表中。
最后,我们可以使用 linprog
解决转换后的问题。
>>> import numpy as np
>>> from scipy.optimize import linprog
>>> c = np.array([-29.0, -45.0, 0.0, 0.0])
>>> A_ub = np.array([[1.0, -1.0, -3.0, 0.0],
... [-2.0, 3.0, 7.0, -3.0]])
>>> b_ub = np.array([5.0, -10.0])
>>> A_eq = np.array([[2.0, 8.0, 1.0, 0.0],
... [4.0, 4.0, 0.0, 1.0]])
>>> b_eq = np.array([60.0, 60.0])
>>> x0_bounds = (0, None)
>>> x1_bounds = (0, 5.0)
>>> x2_bounds = (-np.inf, 0.5) # +/- np.inf can be used instead of None
>>> x3_bounds = (-3.0, None)
>>> bounds = [x0_bounds, x1_bounds, x2_bounds, x3_bounds]
>>> result = linprog(c, A_ub=A_ub, b_ub=b_ub, A_eq=A_eq, b_eq=b_eq, bounds=bounds)
>>> print(result.message)
The problem is infeasible. (HiGHS Status 8: model_status is Infeasible; primal_status is None)
结果表明我们的问题不可行,这意味着没有满足所有约束的解向量。这并不一定意味着我们做错了什么;某些问题确实是不可行的。但是,假设我们决定 \(x_1\) 的边界约束太紧,并且可以放宽到 \(0 \leq x_1 \leq 6\)。在调整我们的代码 x1_bounds = (0, 6)
以反映更改并再次执行后
>>> x1_bounds = (0, 6)
>>> bounds = [x0_bounds, x1_bounds, x2_bounds, x3_bounds]
>>> result = linprog(c, A_ub=A_ub, b_ub=b_ub, A_eq=A_eq, b_eq=b_eq, bounds=bounds)
>>> print(result.message)
Optimization terminated successfully. (HiGHS Status 7: Optimal)
结果表明优化成功。我们可以检查目标值(result.fun
)是否与 \(c^Tx\) 相同
>>> x = np.array(result.x)
>>> obj = result.fun
>>> print(c @ x)
-505.97435889013434 # may vary
>>> print(obj)
-505.97435889013434 # may vary
我们还可以检查所有约束是否在合理的公差范围内得到满足
>>> print(b_ub - (A_ub @ x).flatten()) # this is equivalent to result.slack
[ 6.52747190e-10, -2.26730279e-09] # may vary
>>> print(b_eq - (A_eq @ x).flatten()) # this is equivalent to result.con
[ 9.78840831e-09, 1.04662945e-08]] # may vary
>>> print([0 <= result.x[0], 0 <= result.x[1] <= 6.0, result.x[2] <= 0.5, -3.0 <= result.x[3]])
[True, True, True, True]
分配问题#
线性总和分配问题示例#
考虑为游泳混合泳接力队选择学生的问题。我们有一个表格,显示了五名学生每种游泳方式的时间
学生 |
仰泳 |
蛙泳 |
蝶泳 |
自由泳 |
---|---|---|---|---|
A |
43.5 |
47.1 |
48.4 |
38.2 |
B |
45.5 |
42.1 |
49.6 |
36.8 |
C |
43.4 |
39.1 |
42.1 |
43.2 |
D |
46.5 |
44.1 |
44.5 |
41.2 |
E |
46.3 |
47.8 |
50.4 |
37.2 |
我们需要为四种游泳方式中的每一种选择一名学生,以使总接力时间最小化。这是一个典型的线性总和分配问题。我们可以使用 linear_sum_assignment
来解决它。
线性总和分配问题是最著名的组合优化问题之一。给定一个“成本矩阵” \(C\),问题是选择
每行恰好一个元素
不从任何列中选择多个元素
这样选择的元素之和最小化
换句话说,我们需要将每一行分配给一列,以使相应条目的总和最小化。
形式上,设 \(X\) 为布尔矩阵,其中 \(X[i,j] = 1\) 当且仅当第 \(i\) 行被分配给第 \(j\) 列时。那么最优分配的成本为
第一步是定义成本矩阵。在此示例中,我们希望将每种游泳方式分配给一名学生。linear_sum_assignment
能够将成本矩阵的每一行分配给一列。因此,为了形成成本矩阵,需要转置上表,以便行对应于游泳方式,列对应于学生
>>> import numpy as np
>>> cost = np.array([[43.5, 45.5, 43.4, 46.5, 46.3],
... [47.1, 42.1, 39.1, 44.1, 47.8],
... [48.4, 49.6, 42.1, 44.5, 50.4],
... [38.2, 36.8, 43.2, 41.2, 37.2]])
我们可以使用 linear_sum_assignment
解决分配问题
>>> from scipy.optimize import linear_sum_assignment
>>> row_ind, col_ind = linear_sum_assignment(cost)
row_ind
和 col_ind
是成本矩阵的最佳分配矩阵索引
>>> row_ind
array([0, 1, 2, 3])
>>> col_ind
array([0, 2, 3, 1])
最佳分配是
>>> styles = np.array(["backstroke", "breaststroke", "butterfly", "freestyle"])[row_ind]
>>> students = np.array(["A", "B", "C", "D", "E"])[col_ind]
>>> dict(zip(styles, students))
{'backstroke': 'A', 'breaststroke': 'C', 'butterfly': 'D', 'freestyle': 'B'}
最佳混合泳总时间为
>>> cost[row_ind, col_ind].sum()
163.89999999999998
请注意,此结果与每种游泳方式的最小时间之和不同
>>> np.min(cost, axis=1).sum()
161.39999999999998
因为学生“C”是“蛙泳”和“蝶泳”风格中最好的游泳者。我们不能将学生“C”分配给这两种风格,因此我们将学生 C 分配给“蛙泳”风格,将 D 分配给“蝶泳”风格,以最大程度地缩短总时间。
参考文献
一些进一步的阅读和相关软件,例如 Newton-Krylov [KK],PETSc [PP] 和 PyAMG [AMG]
D.A. Knoll 和 D.E. Keyes,“无雅可比牛顿-克雷洛夫方法”,J. Comp. Phys. 193, 357 (2004)。 DOI:10.1016/j.jcp.2003.08.010
PETSc https://www.mcs.anl.gov/petsc/ 及其 Python 绑定 https://bitbucket.org/petsc/petsc4py/
PyAMG(代数多重网格预处理器/求解器) pyamg/pyamg#issues
混合整数线性规划#
背包问题示例#
背包问题是一个众所周知的组合优化问题。给定一组物品,每个物品都有大小和价值,问题是在总大小低于某个阈值的情况下选择最大化总价值的物品。
形式上,让
令 \(x_i\) 为一个布尔变量,表示物品 \(i\) 是否包含在背包中,
令 \(n\) 为物品的总数,
令 \(v_i\) 为物品 \(i\) 的价值,
令 \(s_i\) 为物品 \(i\) 的大小,以及
令 \(C\) 为背包的容量。
那么问题是
尽管目标函数和不等式约束在决策变量 \(x_i\) 中是线性的,但这与典型的线性规划问题不同,因为决策变量只能取整数值。 具体来说,我们的决策变量只能是 \(0\) 或 \(1\),因此这被称为二元整数线性规划(BILP)。 这个问题属于更大的混合整数线性规划(MILP)类别,我们可以使用 milp
来解决。
在我们的例子中,有 8 个物品可供选择,每个物品的大小和价值如下所示。
>>> import numpy as np
>>> from scipy import optimize
>>> sizes = np.array([21, 11, 15, 9, 34, 25, 41, 52])
>>> values = np.array([22, 12, 16, 10, 35, 26, 42, 53])
我们需要约束我们的八个决策变量为二进制。 我们通过添加一个 Bounds
约束来确保它们介于 \(0\) 和 \(1\) 之间,并应用“整数性”约束来确保它们要么是 \(0\) 要么是 \(1\)。
>>> bounds = optimize.Bounds(0, 1) # 0 <= x_i <= 1
>>> integrality = np.full_like(values, True) # x_i are integers
背包容量约束使用 LinearConstraint
指定。
>>> capacity = 100
>>> constraints = optimize.LinearConstraint(A=sizes, lb=0, ub=capacity)
如果我们遵循线性代数的通常规则,输入 A
应该是一个二维矩阵,下限和上限 lb
和 ub
应该是一维向量,但是 LinearConstraint
很宽容,只要输入可以广播到一致的形状即可。
使用上面定义的变量,我们可以使用 milp
解决背包问题。 请注意,milp
会最小化目标函数,但我们希望最大化总价值,因此我们将 c 设置为价值的负数。
>>> from scipy.optimize import milp
>>> res = milp(c=-values, constraints=constraints,
... integrality=integrality, bounds=bounds)
让我们检查一下结果
>>> res.success
True
>>> res.x
array([1., 1., 0., 1., 1., 1., 0., 0.])
这意味着我们应该选择物品 1、2、4、5、6,以在大小约束下优化总价值。 请注意,这与我们解决线性规划松弛(没有整数约束)并尝试舍入决策变量所获得的结果不同。
>>> from scipy.optimize import milp
>>> res = milp(c=-values, constraints=constraints,
... integrality=False, bounds=bounds)
>>> res.x
array([1. , 1. , 1. , 1. ,
0.55882353, 1. , 0. , 0. ])
如果我们把这个解向上舍入到 array([1., 1., 1., 1., 1., 1., 0., 0.])
,我们的背包将超出容量限制,而如果我们向下舍入到 array([1., 1., 1., 1., 0., 1., 0., 0.])
,我们将得到一个次优解。
有关更多 MILP 教程,请参阅 SciPy Cookbooks 上的 Jupyter 笔记本